In the ever-evolving world of agriculture, leveraging technology to optimize yield has become more than a trend; it’s a necessity. This is where Harvest Prediction Algorithms come into play, revolutionizing farming practices on a global scale. This comprehensive guide aims to shed light on these groundbreaking algorithms, providing insight into how they can be used to predict crop yield with impressive accuracy.
So, whether you’re a seasoned farmer looking to modernize your operations, a budding agritech enthusiast, or a tech-savvy individual interested in the intersection of agriculture and technology, this guide will equip you with valuable knowledge about Harvest Prediction Algorithms. Sit back, read on, and prepare to delve into the fascinating world of predictive farming.
Table of Contents
Understanding Harvest Prediction
Understanding the Intricacies of Harvest Prediction Algorithms The world of agriculture has historically been rooted in manual labor, intuition, and experience. However, the advent of technology has ushered in an era of precision farming, where data-driven insights and algorithms are revolutionizing the farming landscape. At the heart of this revolution lies the intriguing world of harvest prediction algorithms.
Harvest prediction algorithms, as the name suggests, are intelligent models that predict crop yield. They are the crystal ball of the modern farmer, providing insights on when and how much a farm will produce at a given time. These algorithms are not pulled out of thin air but are meticulously designed using various statistical and machine-learning techniques.
The beauty of these algorithms lies in their ability to analyze complex patterns from various data sources, such as weather conditions, soil health, crop type, and historical yield data. They then use this information to predict future harvests accurately. Moreover, these algorithms are continually learning and improving.
As more data is fed into them, their predictions become increasingly accurate. This continuous learning process makes them an invaluable tool for farmers, helping them plan their harvesting schedules, manage resources efficiently, and ultimately improve their bottom line. In essence, harvest prediction algorithms are the unsung heroes of modern farming.
They work silently in the background, crunching numbers and analyzing patterns, all in an effort to bring certainty in an industry often at the mercy of unpredictable elements. It’s not magic, but it’s certainly a clever blend of science, technology, and farming expertise.
Definition and Importance
Harvest prediction algorithms, the cutting-edge technology in the agricultural sector, are game-changers that transcend traditional farming methods. These sophisticated algorithms are designed to predict the size and timing of harvests with remarkable precision, thereby enabling farmers to optimize their yield. By leveraging data analysis and machine learning, these algorithms can significantly streamline farming operations, reduce wastage, and boost profitability.
The importance of harvest prediction algorithms lies in their potential to revolutionize agricultural practices, promoting sustainability and efficiency in an industry that is fundamental to human survival and prosperity.
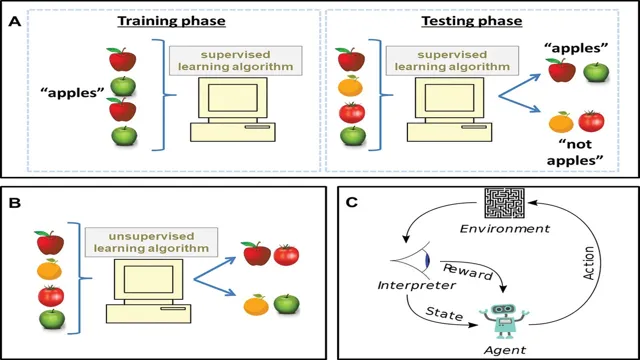
Role in Agriculture
“Harvest prediction algorithms, the unsung heroes in the agricultural sector, are revolutionizing farming practices globally. These sophisticated, data-driven models accurately forecast crop yields, empowering farmers to plan their harvesting and marketing strategies effectively. This fusion of traditional farming with cutting-edge technology not only increases profitability but also ensures food security.
So, let’s delve deeper into the fascinating world of harvest prediction algorithms, where silicon meets soil, redefining the agricultural landscape.”
Types of Prediction Algorithms
Prediction algorithms are the heartbeat of the data science revolution, underlying the power of artificial intelligence and machine learning. They are the silent wizards, predicting everything from the stock market’s next moves to the weather for your weekend getaway. But, let’s turn our attention to a specific application – harvest prediction algorithms.
Harvest prediction algorithms are a subset of prediction algorithms that are designed to predict the yield of a harvest. They are becoming increasingly popular in the agricultural sector, enabling farmers to make more informed decisions about when to plant, irrigate, and harvest their crops. There are several types of harvest prediction algorithms, each with its unique approach.
Linear regression algorithms, for instance, use historical crop yield data to predict future yields. Decision tree algorithms, on the other hand, predict yields based on a set of decision rules. Meanwhile, neural network algorithms mimic the human brain’s functioning to forecast harvest yields.
Another type is the time series algorithm, designed to analyze data points collected over time to predict future results. We also have ensemble algorithms that combine predictions from multiple algorithms to produce a final prediction. Each of these algorithms has its strengths and weaknesses, and the choice of algorithm depends on the specific needs of the farmer and the nature of the data available.
In essence, harvest prediction algorithms are like the oracle for farmers, offering predictions to guide their crucial farming decisions. They are not just algorithms; they are the crystal balls of modern agriculture.
Machine Learning Models
In the fascinating world of agriculture, harvest prediction algorithms, a type of machine learning model, have emerged as a game-changer. These algorithms, powered by cutting-edge technology, are designed to analyze vast amounts of data, from weather patterns to soil conditions, to predict harvest yields accurately. The result of this unprecedented innovation? Farmers can better plan their strategies, minimize losses, and maximize productivity.
In essence, these algorithms are revolutionizing farming practices, making agriculture smarter and more efficient than ever before. So, let’s delve deeper into the world of machine learning models and their role in shaping the future of agriculture.
Statistical Models
Harnessing the Power of Statistical Models: A Closer Look at Harvest Prediction Algorithms In the ever-evolving world of agri-tech, harvest prediction algorithms have emerged as a game changer. By leveraging the power of statistical models, these intricate algorithms can accurately forecast crop yields, enabling farmers to optimize their harvest strategies. They delve deep into historical weather data, crop growth patterns, and soil conditions, conducting a data-driven symphony that culminates in precise predictions.
This innovative technology not only revolutionizes farming practices but also paves the way for a sustainable future where data-driven decisions can mitigate potential risks and maximize agricultural productivity.
Hybrid Models
In the realm of agricultural technology, hybrid models are emerging as game-changers, particularly where harvest prediction algorithms are concerned. These innovative tools blend various forecasting techniques to yield highly accurate harvest predictions. With their capacity to analyze complex data from diverse sources, hybrid models are revolutionizing the agricultural sector’s approach to productivity and sustainability.
The blend of traditional knowledge and modern analytics is not just a testament to technological advancement but also a witty and clever solution to age-old farming challenges. The future of farming is here, and it’s wrapped in the brilliance of hybrid models and harvest prediction algorithms.
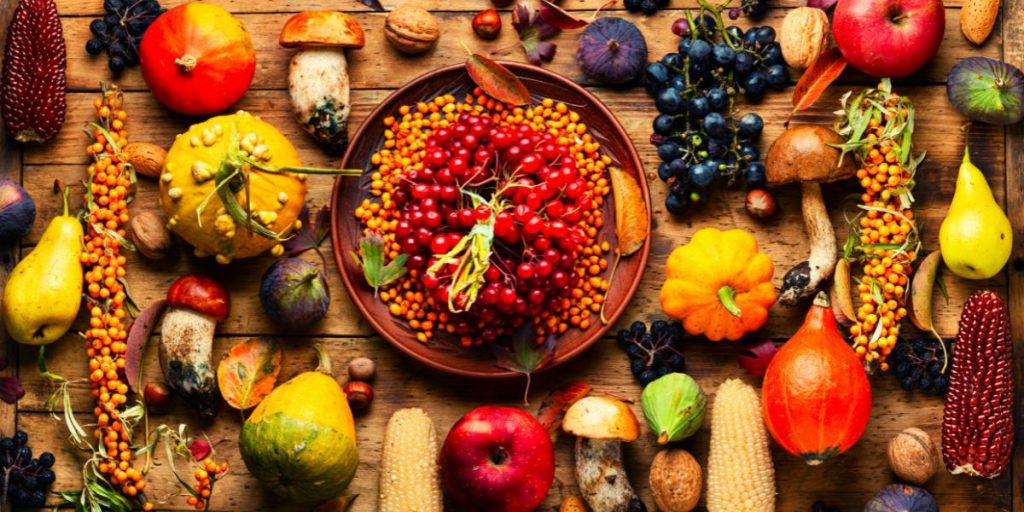
Implementing Prediction Algorithms
Sowing the Seeds of Success In the digital age, the agriculture industry is increasingly turning to technology to optimize production and efficiency. One of the most intriguing advancements in this field is the rise of harvest prediction algorithms. These algorithms are essentially sets of rules or instructions that are designed to analyze patterns, trends, and relationships within a particular set of data.
In the context of agriculture, these algorithms can analyze various factors such as weather patterns, soil conditions, and crop growth rates to predict the potential yield of a harvest. Implementing these harvest prediction algorithms can be a game-changer for farmers and agricultural businesses. For instance, by accurately predicting the yield of a harvest, farmers can better plan their planting and harvesting schedules, manage their resources more effectively, and ultimately, increase their profits.
Moreover, these algorithms can also play a crucial role in addressing global food security issues. By providing accurate and timely predictions, they can help ensure that food production keeps pace with the growing global population. However, implementing these algorithms is not without its challenges.
It requires a deep understanding of both data analysis and agricultural practices. Additionally, the accuracy of these predictions is heavily dependent on the quality and quantity of data available. Therefore, it is crucial to invest in reliable data collection and management systems.
Data Collection and Processing
In the dynamic realm of agriculture, harvest prediction algorithms are becoming increasingly significant. These ingenious computational models utilize various data collected, such as weather patterns, soil conditions, and crop growth rates, to forecast future harvest yields. The collection and processing of this data is a vital step in enhancing the precision of these algorithms.
This information not only leads to more accurate predictions but also aids in making informed decisions on crop management, ultimately optimizing productivity. So, let’s delve into the fascinating world of data collection and processing in the context of harvest prediction algorithms.
Model Training and Evaluation
Harnessing the power of harvest prediction algorithms, we delve into the fascinating realm of model training and evaluation. This critical process involves teaching the algorithm to discern patterns, trends, and relationships within a dataset and then testing its predictive accuracy. The magic lies in its ability to foresee agricultural yields, enabling farmers to manage resources and maximize productivity optimally.
So, let’s embark on this intriguing journey of model training and evaluation, where algorithms and data dance together to orchestrate the symphony of bountiful harvests.
Real-world Case Studies
Real-world Case Studies of Harvest Prediction Algorithms In the dynamic sphere of agriculture, technology has emerged as an ally, providing farmers with tools to predict, plan, and prosper. Among these tools, harvest prediction algorithms have revolutionized the way we approach farming. Let’s delve into some real-world case studies that spotlight the effectiveness and impact of these algorithms.
In the vineyards of California, algorithms have been employed to predict grape yields with remarkable accuracy. Winemakers are now able to plan their production schedules and marketing strategies way ahead of the harvest season, leading to enhanced efficiency and profitability. In another instance, potato farmers in Idaho have benefitted from harvest prediction algorithms.
They have been able to determine the optimum time for harvest, minimizing waste and maximizing yield. This has not only increased their revenue but also contributed to sustainable farming practices. Across the Atlantic, olive growers in Spain have also seen the advantages of these predictive tools.
With the ability to anticipate crop yield, they have been able to manage their resources better, reducing costs and increasing their bottom line. These case studies underline the transformative potential of harvest prediction algorithms. By harnessing data and technology, farmers around the world are reaping the benefits of accurate forecasting, making agriculture a more predictable and profitable venture.
With the continued advancement in technology, the future of farming looks promising, with harvest prediction algorithms leading the way.
Success in Crop Yield Forecasting
Harvest prediction algorithms are revolutionizing the agricultural sector, transforming the way crop yields are anticipated. These innovative models leverage machine learning and AI to analyze a myriad of factors influencing crop output – from weather patterns to soil quality. This groundbreaking technology not only offers a significant boost to crop yield forecasting accuracy but also empowers farmers to make data-driven decisions.
Consequently, the agricultural sector is witnessing a paradigm shift, where the precision of predictive analytics is steadily replacing reliance on intuition. With harvest prediction algorithms, the future of farming appears as bountiful as the crops they forecast.
Challenges and Solutions
Navigating the labyrinth of harvest prediction algorithms is no easy feat, with its fair share of challenges that can bewilder even the most seasoned farmers. From dealing with intricate data sets to battling unpredictable weather patterns, it’s a roller-coaster ride that requires nimble adaptability. However, with the right blend of technology and expert insights, these obstacles can morph into opportunities.
Leveraging machine learning and AI can offer accurate predictions, aiding in informed decision-making. Thus, despite the challenges, innovative solutions in the form of advanced harvest prediction algorithms can transform agriculture into a streamlined, efficient, and productive industry.
Conclusion
Harvest prediction algorithms can be likened to the weatherman of agriculture. Just as we depend on weather forecasts to plan our day, farmers can utilize these algorithms to predict and plan their harvest. These are not just mathematical models running numbers but more like crystal balls that provide insights into the future of farming.
They offer a clever mix of data analysis and agricultural science, helping farmers maximize yield and minimize loss. Therefore, it’s safe to say that these algorithms are not just sowing seeds of prediction but reaping the benefits of technology for a more fruitful future in agriculture.
Frequently Asked Questions [FAQs]
1. What are harvest prediction algorithms?
Harvest prediction algorithms are computational models used to predict the yield of a particular crop for a particular season. They use various parameters like weather data, soil type, crop type, and other agronomic factors to make these predictions.
2. How do harvest prediction algorithms work?
Harvest prediction algorithms work by processing huge amounts of data related to weather conditions, soil type, crop type, historical yield data, and other agronomic factors. They use machine learning techniques to identify patterns and trends in the data, which are then used to predict future crop yields.
3. Why are harvest prediction algorithms important?
Harvest prediction algorithms can help farmers make informed decisions about their farming practices. By predicting the yield, farmers can plan their harvest and post-harvest activities more efficiently. These algorithms can also help in mitigating risks associated with climate change and other unpredictable factors.
4. What are the limitations of harvest prediction algorithms?
While harvest prediction algorithms can be quite accurate, they do have certain limitations. They rely heavily on the quality and quantity of data. If the data is not accurate or comprehensive enough, the predictions can be off. Moreover, these algorithms may not be able to fully account for unexpected events like sudden weather changes or pest attacks.
5. Can harvest prediction algorithms be used for all types of crops?
Yes, theoretically, harvest prediction algorithms can be used for any crop. However, the accuracy of the predictions might vary depending on the amount of available data and the specific characteristics of each crop. More research and data are needed to improve the algorithms for different types of crops.
Leave a Reply